From Data to Insight
ABOUT US
CALL
HORIZON-CL4-2023-HUMAN-01-CNECT
DURATION
01 January 2024
► 30 June 2027
Grant Agreement ID
101135826
Horizon Europe RIA Project
Today, Artificial Intelligence (AI) has paved a long way since its inception and has started experiencing exponential growth across various industries and shaping our world in ways that were once thought impossible. As AI transitions from research to deployment, leveraging the appropriate data to develop and evaluate AI models has evolved into one of its greatest challenges. Data are in fact the raw material and the most indispensable asset fuelling much of today’s progress in AI, generating previously unattainable insights, assisting more evidence-based decision-making, and bringing tangible business/economic benefits and innovation to all involved stakeholders. However, despite their instrumental role in determining performance, fairness, and robustness of AI systems, data are paradoxically characterised as the most under-valued and de-glamorised aspect of AI while a data-centric focus is typically lacking in the current AI research.
AI-DAPT aims to deliver an innovative and impactful research agenda that will provide tangible benefits to a variety of stakeholders that struggle with making AI services. Seeking to reinstate the pure data-related work in its rightful place, and reinforcing the generalizability, reliability, trustworthiness, and fairness of Al solutions, AI-DAPT vision relies on the implementation of an AIOps framework to support and automate AI pipelines that continuously learn and adapt based on their context. It enables proper purposing, collection, documentation, (bias) valuation, annotation, curation and synthetic generation of data, while keeping humans-in-the-loop across five axis: (i) Data Design for AI, (ii) Data Nurturing for AI, (iii) Data Generation for AI, (iv) Model Delivery for AI, (v) Data-Model Optimization for AI.
AI-DAPT brings forward a two-fold data-centric mentality in AI:
- Data: AI-driven automation for data pipelines based on Explainable AI (XAI) techniques as well as synthetic data generation and observability.
- Model: Automation on AI model building and hybrid science-AI solutions, bringing together data-driven AI models and science-based (first-principles) models that build on high-quality data.
Bridging the gap between data-centric and model-centric AI, AI-DAPT will turn over a new leaf in trustworthy AI and will nurture an ecosystem involving all AI and data value-chain stakeholders. The aim is to enhance their prosperous collaboration in order to deliver and apply innovative AI-driven methods that rely on smart and dynamic end-to-end automation of data, AI training/inference pipelines in the cloud-edge computing continuum.
To demonstrate the actual innovation and added value that can be derived through the AI-DAPT scientific advancements, the AI-DAPT results will be validated in two ways:
- By applying them to tackle real-world challenges in four key industries: (4) Health, Robotics, Energy, and Manufacturing.
- By integrating them into various AI solutions, whether open source or commercial, already present in the market.
The AI-DAPT CONCEPT
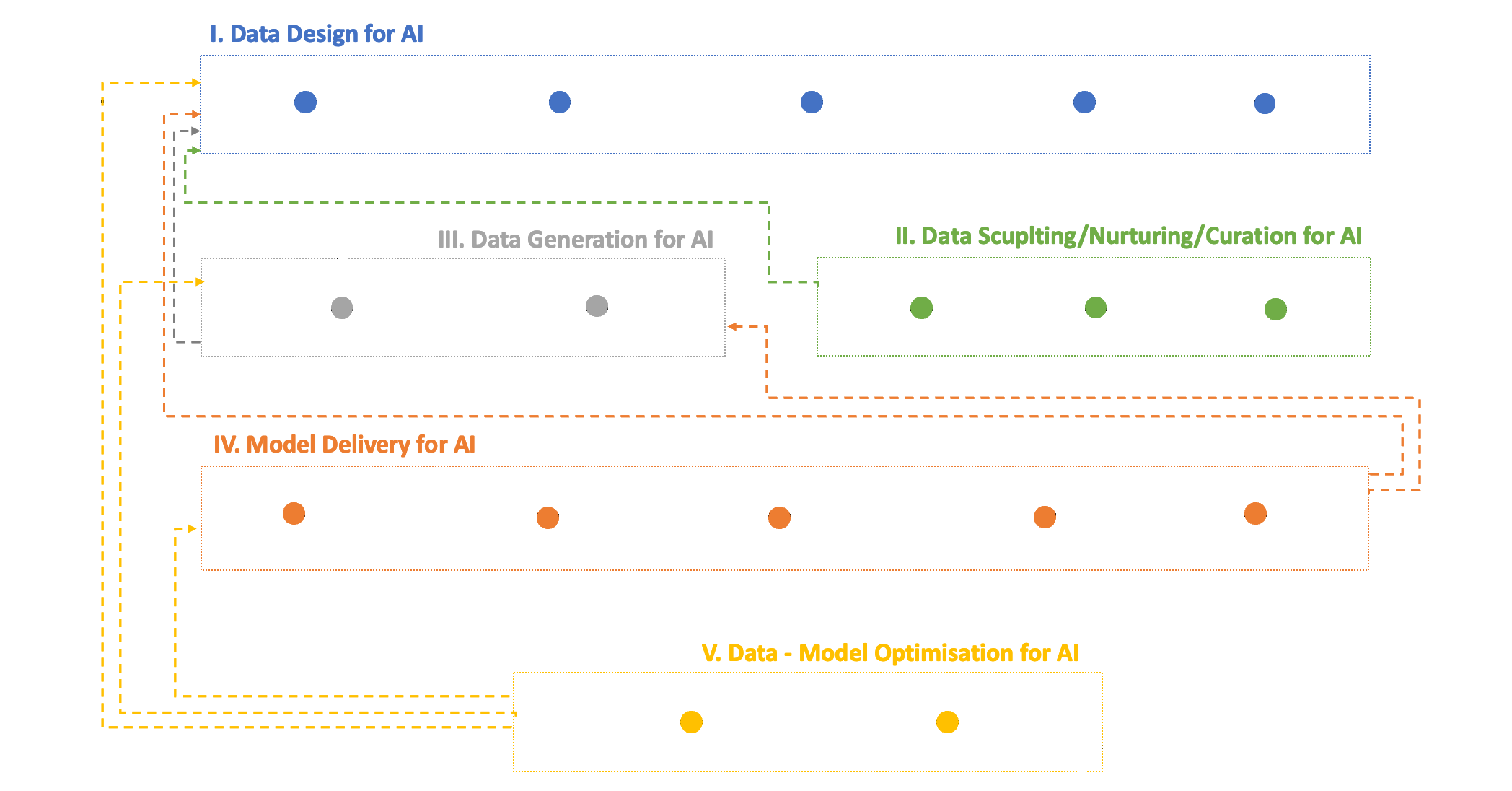
Figure – AI-DAPT Concept of data-AI pipelines across their lifecycle
AI-DAPT approaches AI with a focus on data, leveraging automation and AI techniques to construct robust, intelligent, and scalable data-AI pipelines. These pipelines are designed to continuously adapt and learn from their environment, executing efficient steps that integrate operational and business logic. They can be triggered by schedules, real-time events, or other triggers, and can run in parallel or sequence.
During Phase I, known as “Data Design for AI“, data scientists select suitable data for the AI solution, drawing on domain knowledge. Automated processes fetch raw data from internal databases to ensure it’s up-to-date. Data characteristics are analyzed and summarized collaboratively by data scientists and business users, documenting findings for standardized reports.
In Phase II, “Data Sculpting/Nurturing/Curation for AI“, AI/ML techniques are employed to ensure data representativeness and quality. Features are annotated semantically and engineered, with relevant ones chosen for the AI model. Cleaning techniques are applied to enhance data quality.
Phase III, “Data Generation for AI“, tackles data scarcity by creating synthetic data to supplement or replace real data. Data utility assessment evaluates the suitability of synthetic data.
In Phase IV, “Model Delivery for AI“, data scientists oversee the AI model lifecycle, using hybrid science-guided ML approaches. Models are configured, trained, and deployed for real-world application, considering prediction uncertainty.
Phase V, “Data-Model Optimization for AI“, focuses on continuous monitoring and improvement of the AI solution based on real-life operation circumstances. Data and model observability ensure timely adjustments.
Throughout the process, Explainable AI techniques elucidate AI models and results, filtering out low-quality or biased data. The pipeline combines manual and automated steps, ensuring efficiency and reliability in AI development and deployment.